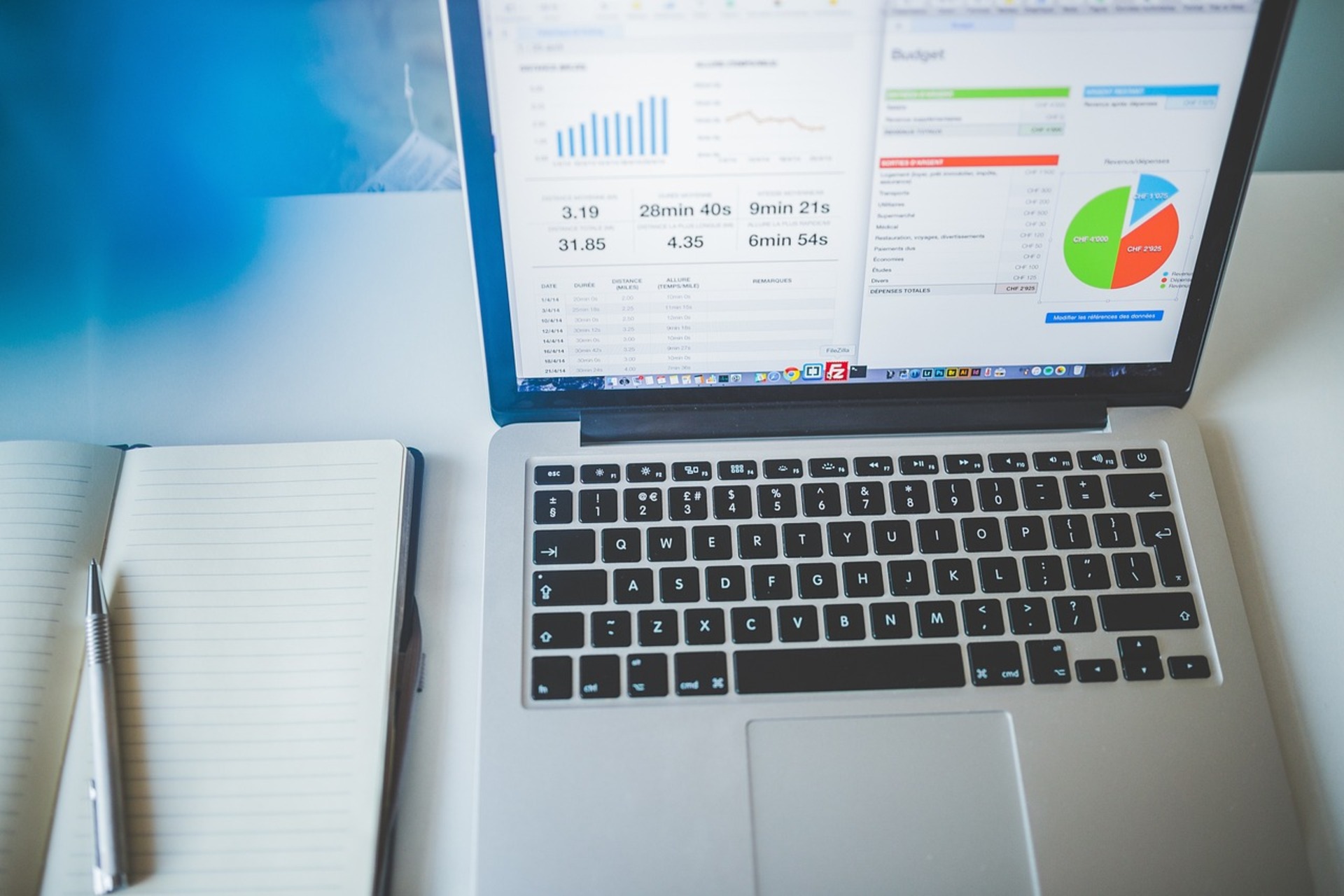
This proactive approach is crucial in today’s data-driven landscape, where organizations handle vast amounts of sensitive information.
At the core of predictive analytics is the analysis of historical data to forecast future events. In the context of risk management, this involves identifying patterns and anomalies that could signify potential threats. By examining past data breaches, unauthorized access attempts, and other security incidents, predictive models can determine the likelihood of similar events occurring in the future.
One significant application is in fraud detection. Financial institutions, for example, use predictive analytics to monitor transactions in real time, flagging unusual activities that deviate from established patterns. This allows for immediate action to prevent fraudulent activities, thereby safeguarding both the institution and its customers.
Another critical area is cybersecurity. Predictive analytics can help in identifying vulnerabilities within a network by analyzing logs and user behavior. By predicting where and how attacks might occur, organizations can reinforce their defenses accordingly. This is particularly important for preventing data breaches, which can have severe financial and reputational consequences.
Moreover, predictive analytics aids in compliance management. Regulations such as GDPR and HIPAA impose stringent requirements on data handling and protection. Predictive tools can ensure ongoing compliance by forecasting potential compliance issues and allowing organizations to address them proactively.
The integration of predictive analytics into data risk management also improves decision-making. By providing a data-driven basis for risk assessment, it enables more accurate and timely decisions. This can be crucial in situations requiring immediate action to mitigate risks, such as in incident response and disaster recovery planning.
However, implementing predictive analytics in data risk management is not without challenges. It requires substantial investment in technology and skilled personnel. Additionally, the quality of predictions heavily depends on the quality of the data used. Organizations must ensure their data is accurate, complete, and up-to-date to maximize the effectiveness of predictive models.
Predictive analytics represents a transformative approach in data risk management, offering a proactive stance against potential threats. By forecasting risks and enabling preemptive actions, it not only protects sensitive information but also supports regulatory compliance and enhances overall security posture.
WWW.BARETZKY.NET