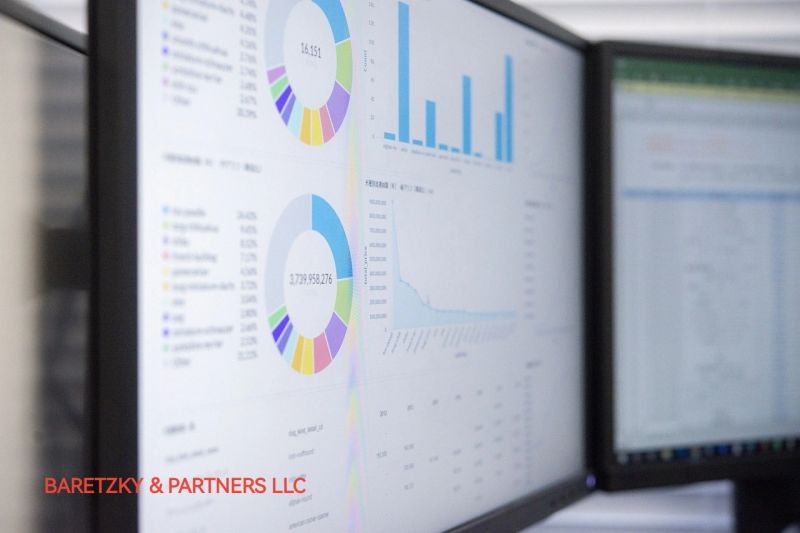
It involves continuous assessment and validation of data against predefined standards, identifying discrepancies, and taking corrective actions to maintain data integrity.
At its core, data quality monitoring revolves around several key dimensions: accuracy, completeness, consistency, timeliness, and relevance. Accuracy ensures that the data reflects real-world conditions accurately. Completeness checks if all necessary data fields are populated, while consistency ensures that the data does not contain contradictions. Timeliness verifies that the data is up-to-date, and relevance ensures that the data is suitable for its intended use.
Effective data quality monitoring often employs automated tools that regularly scan data sets, flagging anomalies like missing values, duplicates, or outdated entries. These tools can provide dashboards and reports to help data stewards and analysts quickly identify and address issues.
Moreover, data quality monitoring should be an ongoing process, integrated into the organization’s data governance framework. It requires collaboration between IT, data management teams, and business units to ensure that data remains a reliable asset. As organizations increasingly rely on data-driven decision-making, maintaining high data quality through diligent monitoring becomes even more essential for achieving business objectives.
WWW.BARETZKY.NET