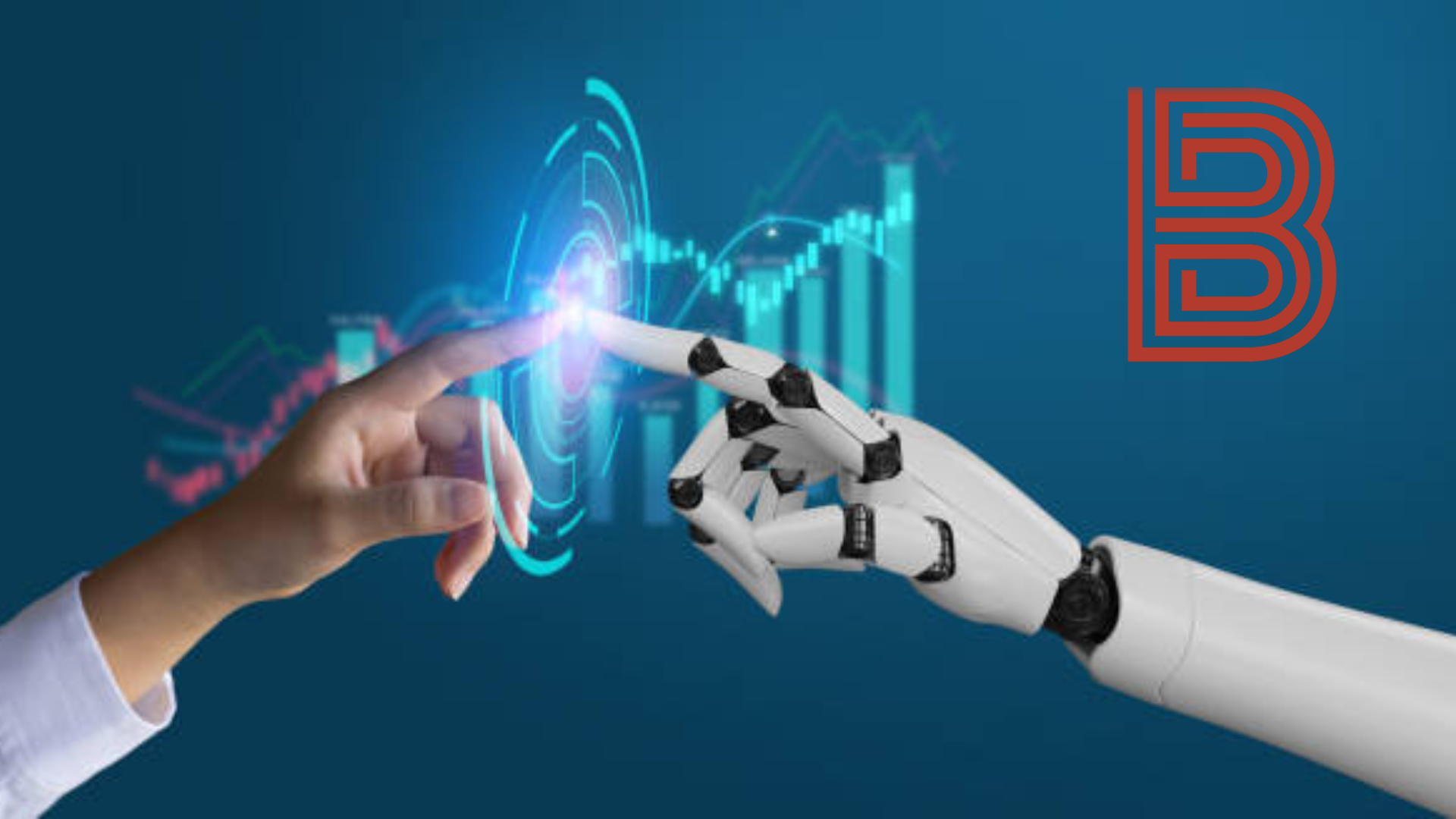
However, the integration of AI brings unique challenges, particularly in reputation risk management. Reputation risk—defined as potential damage to an organization’s credibility or public trust—can escalate quickly when AI systems fail, behave unethically, or generate unintended consequences.
One significant risk stems from algorithmic bias. AI systems trained on biased or incomplete data can produce discriminatory outcomes, affecting marginalized groups and harming an organization’s reputation. High-profile cases, such as biased hiring algorithms or facial recognition errors, demonstrate how such incidents can spark public backlash and regulatory scrutiny. Companies must ensure fairness, transparency, and inclusivity in their AI development to mitigate these risks.
Another factor is the lack of transparency or “black-box” nature of some AI models. When stakeholders cannot understand how decisions are made, trust diminishes. For example, if an AI-powered credit system denies loans without clear reasoning, it can lead to accusations of unfairness and erode customer confidence. Organizations should prioritize explainable AI to maintain accountability and public trust.
Data privacy is another area of concern. AI systems often rely on massive amounts of sensitive data. Mismanagement or unauthorized use of this data can lead to reputational damage, legal penalties, and loss of customer trust. Robust data governance frameworks and adherence to privacy regulations like GDPR are essential.
To address these risks, organizations must integrate AI-specific controls into their overall reputation risk management strategies. Regular audits, ethical AI guidelines, stakeholder communication, and incident response plans are vital. By proactively addressing potential issues, companies can harness AI’s benefits while safeguarding their reputation in a rapidly evolving landscape.
WWW.BARETZKY.NET