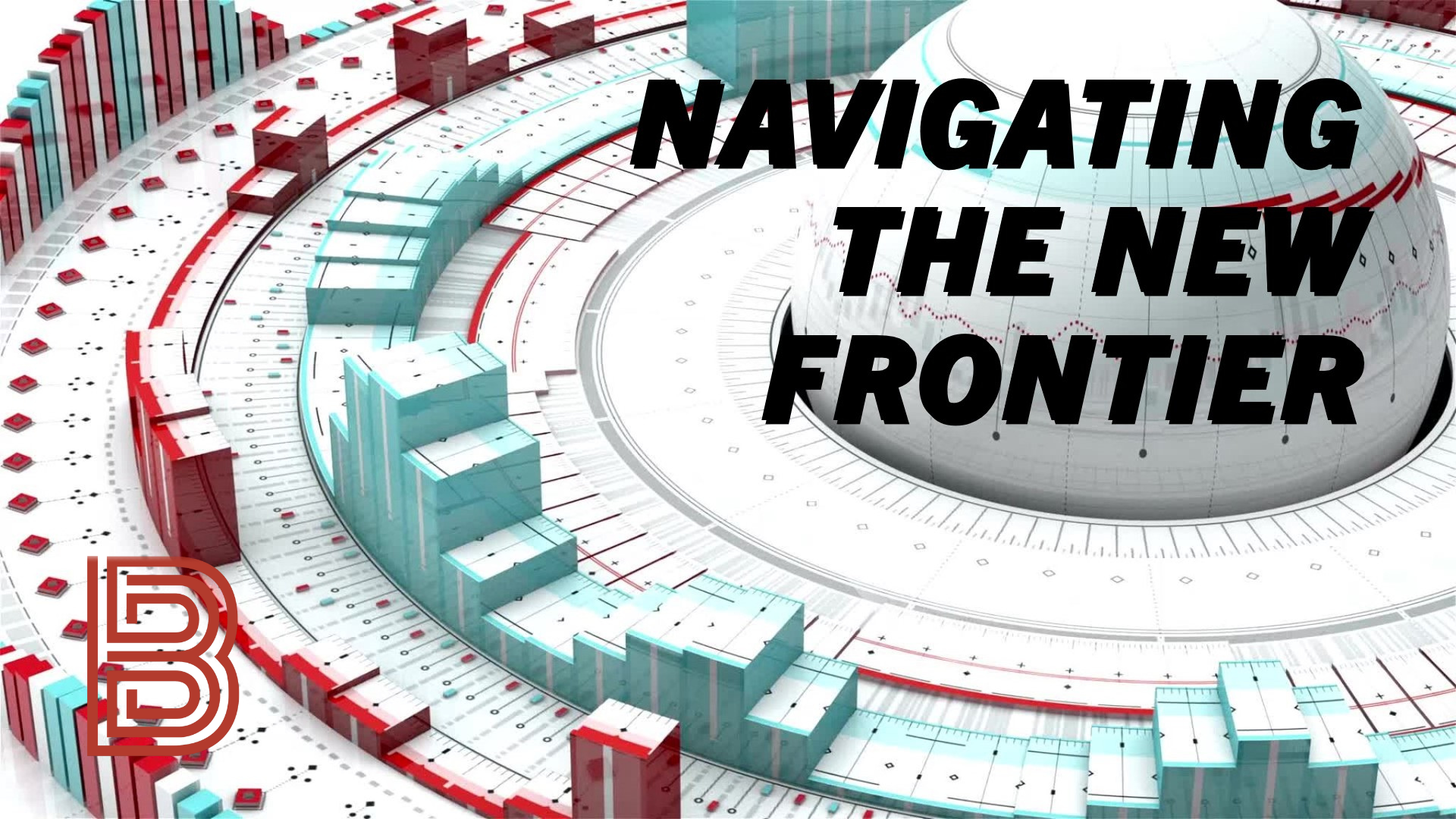
Artificial Intelligence (AI) has rapidly evolved into a transformative force, reshaping industries and redefining traditional practices. In risk management, AI brings both unprecedented opportunities and complex challenges. The integration of AI into risk management processes necessitates robust governance frameworks to ensure ethical, transparent, and effective use. This article explores the multifaceted relationship between AI governance and risk management, detailing key principles, challenges, and best practices to navigate this emerging landscape.
The Role of AI in Risk Management
Risk management involves identifying, assessing, and mitigating potential threats to an organization’s objectives. Traditionally, this domain relied heavily on human expertise and manual processes. AI has introduced a paradigm shift by enabling real-time data analysis, predictive modeling, and automated decision-making. From fraud detection in financial institutions to predictive maintenance in manufacturing, AI-powered tools have enhanced accuracy and efficiency.
Key applications of AI in risk management include:
Predictive Analytics: AI models analyze historical data to forecast potential risks, enabling proactive measures.
Fraud Detection: Machine learning algorithms identify anomalous patterns indicative of fraudulent activity.
Cybersecurity: AI systems detect and respond to cyber threats in real time, reducing response times.
Supply Chain Risk Management: AI monitors global events, weather patterns, and geopolitical risks to optimize supply chain resilience.
Operational Risk: Automation reduces human error and ensures compliance with regulatory requirements.
While the benefits of AI are evident, its adoption also introduces new risks, such as algorithmic bias, data privacy concerns, and systemic vulnerabilities.
Defining AI Governance
AI governance refers to the frameworks, policies, and practices that guide the ethical and responsible use of AI technologies. Effective governance ensures that AI systems align with organizational values, comply with regulatory standards, and mitigate risks associated with their deployment.
Key components of AI governance include:
Ethical Principles: Ensuring fairness, accountability, and transparency in AI applications.
Regulatory Compliance: Adhering to laws and standards such as GDPR, CCPA, and industry-specific guidelines.
Risk Management Frameworks: Integrating AI-specific risks into enterprise risk management (ERM) strategies.
Stakeholder Engagement: Involving diverse stakeholders to address concerns and build trust.
Monitoring and Auditing: Continuously evaluating AI systems for performance, accuracy, and unintended consequences.
Challenges in AI Governance for Risk Management
The intersection of AI governance and risk management presents unique challenges, including:
Algorithmic Bias: AI systems can inadvertently perpetuate biases present in training data, leading to unfair outcomes.
Lack of Transparency: Complex AI models, such as deep learning networks, often operate as “black boxes,” making it difficult to understand their decision-making processes.
Data Privacy and Security: The extensive use of data in AI systems raises concerns about unauthorized access, misuse, and compliance with privacy regulations.
Regulatory Ambiguity: Rapid advancements in AI technology often outpace the development of regulatory frameworks, creating uncertainty for organizations.
Ethical Dilemmas: Balancing innovation with ethical considerations, such as job displacement and societal impact, is a significant challenge.
Best Practices for AI Governance in Risk Management
To effectively integrate AI into risk management, organizations must adopt comprehensive governance strategies. Below are some best practices:
Develop a Clear AI Strategy:
Define objectives and use cases for AI implementation.
Align AI initiatives with organizational goals and risk appetite.
Establish Ethical Guidelines:
Create a code of ethics for AI development and deployment.
Ensure inclusivity and diversity in training data to minimize bias.
Implement Robust Risk Assessment Frameworks:
Identify AI-specific risks, such as model drift and adversarial attacks.
Integrate these risks into broader ERM processes.
Enhance Transparency and Explainability:
Use interpretable models where possible to clarify decision-making processes.
Document AI system behavior and decision rationale.
Strengthen Data Governance:
Enforce strict data access controls and encryption standards.
Regularly audit data quality and compliance with privacy laws.
Engage Stakeholders:
Foster collaboration between technical teams, legal advisors, and business leaders.
Involve external experts and regulators to validate AI practices.
Invest in Continuous Monitoring and Improvement:
Deploy tools for real-time monitoring of AI systems.
Update models and governance frameworks to adapt to evolving risks.
Case Studies in AI Governance and Risk Management
1. Financial Services:
A global bank implemented AI for credit risk assessment but encountered challenges with algorithmic bias. To address this, the bank established a fairness review board, diversified training data, and adopted explainable AI techniques.
2. Healthcare:has rapidly evolved into a transformative force, reshaping industries and redefining traditional practices. In risk management, AI brings both unprecedented opportunities and complex challenges. The integration of AI into risk management processes necessitates robust governance frameworks to ensure ethical, transparent, and effective use. This article explores the multifaceted relationship between AI governance and risk management, detailing key principles, challenges, and best practices to navigate this emerging landscape.
The Role of AI in Risk Management
Risk management involves identifying, assessing, and mitigating potential threats to an organization’s objectives. Traditionally, this domain relied heavily on human expertise and manual processes. AI has introduced a paradigm shift by enabling real-time data analysis, predictive modeling, and automated decision-making. From fraud detection in financial institutions to predictive maintenance in manufacturing, AI-powered tools have enhanced accuracy and efficiency.
Key applications of AI in risk management include:
Predictive Analytics: AI models analyze historical data to forecast potential risks, enabling proactive measures.
Fraud Detection: Machine learning algorithms identify anomalous patterns indicative of fraudulent activity.
Cybersecurity: AI systems detect and respond to cyber threats in real time, reducing response times.
Supply Chain Risk Management: AI monitors global events, weather patterns, and geopolitical risks to optimize supply chain resilience.
Operational Risk: Automation reduces human error and ensures compliance with regulatory requirements.
While the benefits of AI are evident, its adoption also introduces new risks, such as algorithmic bias, data privacy concerns, and systemic vulnerabilities.
Defining AI Governance
AI governance refers to the frameworks, policies, and practices that guide the ethical and responsible use of AI technologies. Effective governance ensures that AI systems align with organizational values, comply with regulatory standards, and mitigate risks associated with their deployment.
Key components of AI governance include:
Ethical Principles: Ensuring fairness, accountability, and transparency in AI applications.
Regulatory Compliance: Adhering to laws and standards such as GDPR, CCPA, and industry-specific guidelines.
Risk Management Frameworks: Integrating AI-specific risks into enterprise risk management (ERM) strategies.
Stakeholder Engagement: Involving diverse stakeholders to address concerns and build trust.
Monitoring and Auditing: Continuously evaluating AI systems for performance, accuracy, and unintended consequences.
Challenges in AI Governance for Risk Management
The intersection of AI governance and risk management presents unique challenges, including:
Algorithmic Bias: AI systems can inadvertently perpetuate biases present in training data, leading to unfair outcomes.
Lack of Transparency: Complex AI models, such as deep learning networks, often operate as “black boxes,” making it difficult to understand their decision-making processes.
Data Privacy and Security: The extensive use of data in AI systems raises concerns about unauthorized access, misuse, and compliance with privacy regulations.
Regulatory Ambiguity: Rapid advancements in AI technology often outpace the development of regulatory frameworks, creating uncertainty for organizations.
Ethical Dilemmas: Balancing innovation with ethical considerations, such as job displacement and societal impact, is a significant challenge.
Best Practices for AI Governance in Risk Management
To effectively integrate AI into risk management, organizations must adopt comprehensive governance strategies. Below are some best practices:
Develop a Clear AI Strategy:
Define objectives and use cases for AI implementation.
Align AI initiatives with organizational goals and risk appetite.
Establish Ethical Guidelines:
Create a code of ethics for AI development and deployment.
Ensure inclusivity and diversity in training data to minimize bias.
Implement Robust Risk Assessment Frameworks:
Identify AI-specific risks, such as model drift and adversarial attacks.
Integrate these risks into broader ERM processes.
Enhance Transparency and Explainability:
Use interpretable models where possible to clarify decision-making processes.
Document AI system behavior and decision rationale.
Strengthen Data Governance:
Enforce strict data access controls and encryption standards.
Regularly audit data quality and compliance with privacy laws.
Engage Stakeholders:
Foster collaboration between technical teams, legal advisors, and business leaders.
Involve external experts and regulators to validate AI practices.
Invest in Continuous Monitoring and Improvement:
Deploy tools for real-time monitoring of AI systems.
Update models and governance frameworks to adapt to evolving risks.
Case Studies in AI Governance and Risk Management
1. Financial Services:
A global bank implemented AI for credit risk assessment but encountered challenges with algorithmic bias. To address this, the bank established a fairness review board, diversified training data, and adopted explainable AI techniques.
2. Healthcare:
A hospital system deployed AI for diagnostic imaging but faced concerns about accuracy and accountability. By integrating rigorous validation protocols and maintaining human oversight, the system improved patient outcomes while mitigating risks.
3. Manufacturing:
A manufacturing firm used AI for predictive maintenance but experienced data breaches. Strengthening data encryption and access controls reduced vulnerabilities and ensured compliance with industry standards.
Regulatory Landscape for AI Governance
Governments and regulatory bodies worldwide are recognizing the need for AI-specific guidelines. Notable initiatives include:
European Union’s AI Act:
Proposes a risk-based framework categorizing AI systems by risk levels (e.g., unacceptable, high, limited).
Emphasizes transparency, accountability, and human oversight.
United States’ AI Bill of Rights:
Outlines principles for ethical AI use, including data privacy and algorithmic fairness.
ISO Standards:
ISO/IEC 38507:2022 provides guidance on governance of AI systems.
Organizations must stay abreast of these developments to ensure compliance and maintain competitive advantage.
Future Trends in AI Governance and Risk Management
As AI continues to evolve, its governance will become increasingly critical. Emerging trends include:
AI Auditing and Certification:
Independent audits and certifications will become standard to verify AI system integrity.
Dynamic Governance Models:
Adaptive frameworks will address the fast-paced nature of AI advancements.
Collaboration Across Sectors:
Public-private partnerships will foster innovation while ensuring responsible AI use.
Focus on Resilience:
Emphasis on building resilient AI systems capable of withstanding adversarial attacks and operational disruptions.
Summary
AI governance is not just a regulatory necessity but a strategic imperative for effective risk management. By embracing ethical principles, robust frameworks, and continuous improvement, organizations can harness the full potential of AI while mitigating its risks. As the landscape of AI and risk management evolves, proactive governance will be the cornerstone of sustainable and responsible innovation.