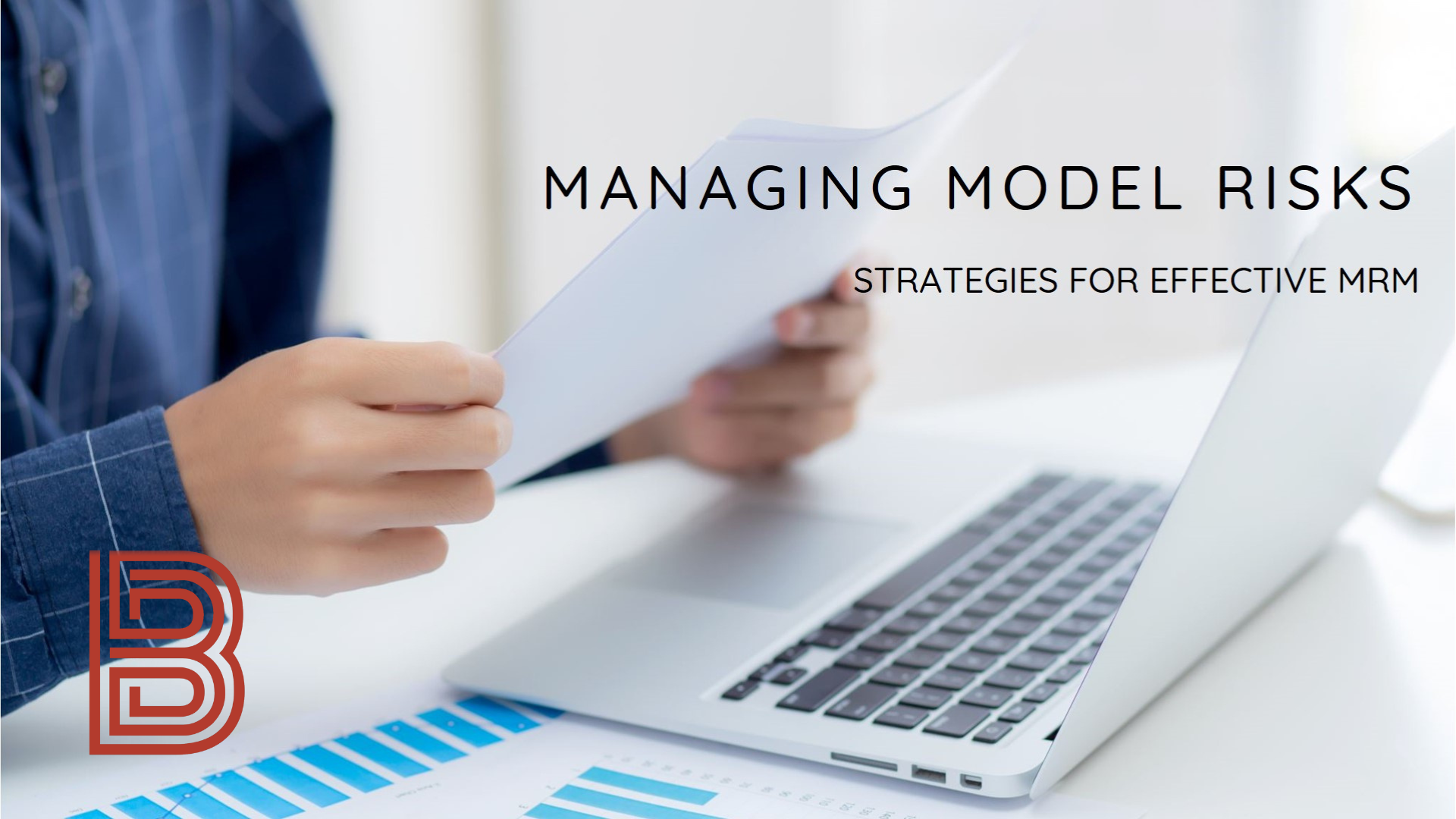
Introduction
In an increasingly data-driven world, models are critical tools in decision-making processes across industries such as finance, healthcare, and technology. However, reliance on models also introduces risks—known as model risks—which can lead to financial losses, reputational damage, regulatory penalties, and operational inefficiencies.
Model Risk Management (MRM) is a discipline aimed at identifying, assessing, mitigating, and monitoring risks associated with the use of models. Effective MRM ensures that models are accurate, reliable, and used appropriately. This paper explores MRM in depth, including its regulatory framework, best practices, governance structures, and emerging trends.
Understanding Model Risk
Model risk arises when a model’s output leads to incorrect or suboptimal decision-making. Model risk can stem from various sources, including:
Data Issues – Poor data quality, biased datasets, or inadequate sample sizes can distort model performance.
Assumption Errors – Models rely on mathematical assumptions that, if incorrect or outdated, can lead to misleading results.
Implementation Errors – Coding mistakes, incorrect parameter calibrations, or improper use of models can introduce errors.
Model Complexity – Overly complex models may be difficult to interpret, validate, and audit.
Evolving Market Conditions – Models built on historical data may fail to adapt to changing economic, financial, or business conditions.
The impact of model risk varies by industry. In financial services, for example, an inaccurate credit risk model may lead to excessive loan defaults, while in healthcare, an erroneous predictive model could affect patient outcomes.
Regulatory Landscape
Regulatory bodies worldwide have recognized the significance of model risk and introduced guidelines for its management. Key regulations and frameworks include:
SR 11-7 (U.S. Federal Reserve and OCC) – This supervisory guidance provides a comprehensive framework for MRM in financial institutions, emphasizing model development, validation, and governance.
Basel III (Bank for International Settlements) – Basel III sets capital adequacy standards that indirectly impact MRM by requiring banks to account for risks, including those arising from models.
European Banking Authority (EBA) Guidelines – The EBA’s guidelines stress the importance of independent model validation and ongoing monitoring.
IFRS 9 (International Financial Reporting Standards) – IFRS 9 requires financial institutions to model expected credit losses, making robust MRM critical for compliance.
General Data Protection Regulation (GDPR) – GDPR has implications for models that process personal data, requiring transparency and fairness in automated decision-making.
Financial institutions, healthcare organizations, and AI-driven businesses must align their MRM practices with these regulatory requirements to avoid penalties and ensure operational stability.
Model Risk Governance
Effective MRM requires a structured governance framework that defines roles, responsibilities, and oversight mechanisms. Key components of model risk governance include:
1. Model Risk Management Framework (MRMF)
A Model Risk Management Framework (MRMF) outlines an organization’s approach to model governance. It typically includes:
Model Definition and Classification – Clear criteria for defining what constitutes a model and its classification based on risk level.
Model Lifecycle Management – Standardized processes for model development, validation, deployment, and retirement.
Risk Appetite and Tolerance – Guidelines on acceptable levels of model risk.
2. Three Lines of Defense (3LoD) Model
A widely adopted governance approach, the Three Lines of Defense model ensures accountability across various stakeholders:
First Line (Model Owners & Developers) – Business units responsible for model development, implementation, and initial validation.
Second Line (Independent Model Validation & Risk Management Teams) – Independent teams that assess model performance and compliance.
Third Line (Internal Audit & External Review) – Auditors who provide independent assurance on the effectiveness of MRM practices.
3. Model Inventory
Maintaining a centralized model inventory helps organizations track all models, their risk ratings, and validation status. This ensures transparency and regulatory compliance.
4. Model Validation and Performance Monitoring
Ongoing model validation is crucial for identifying weaknesses before they lead to significant failures. Key validation activities include:
Conceptual Soundness Review – Assessing underlying assumptions and methodology.
Benchmarking and Backtesting – Comparing model outputs against historical data and alternative models.
Sensitivity Analysis and Stress Testing – Evaluating model behavior under extreme conditions.
Best Practices in Model Risk Management
To build a robust MRM program, organizations should adopt the following best practices:
Clear Model Development Standards – Define standard protocols for data collection, feature selection, algorithm design, and documentation.
Independent Model Validation – Separate model validation from development to avoid conflicts of interest.
Continuous Monitoring – Implement real-time monitoring and periodic reviews to detect model degradation.
Robust Documentation – Maintain comprehensive documentation covering model assumptions, limitations, and validation results.
Stakeholder Training and Awareness – Educate employees on the importance of model risk and their role in mitigating it.
Automated MRM Tools – Utilize advanced software solutions for model inventory management, validation tracking, and risk reporting.
Challenges in Model Risk Management
Despite advancements in MRM, organizations face several challenges:
High Costs and Resource Constraints – Implementing a robust MRM framework requires significant investments in technology and personnel.
Rapid Technological Advancements – Emerging AI and machine learning models introduce complexities in risk assessment.
Data Privacy and Security Concerns – Ensuring model compliance with data protection laws is an ongoing challenge.
Regulatory Complexity – Organizations operating in multiple jurisdictions must navigate diverse regulatory expectations.
Black-Box Models – Highly complex models, such as deep learning algorithms, pose interpretability challenges.
Emerging Trends in Model Risk Management
As industries continue to evolve, MRM is also undergoing transformation. Key trends include:
Artificial Intelligence and Machine Learning (AI/ML) Governance – The rise of AI-driven models necessitates enhanced validation techniques and explainability tools.
RegTech Adoption – Regulatory technology (RegTech) solutions leverage automation to streamline compliance and reporting processes.
Explainable AI (XAI) – Organizations are investing in XAI techniques to enhance model transparency and accountability.
Cloud-Based Model Risk Management – Cloud computing enables real-time model monitoring and collaboration across geographies.
Ethical AI and Fairness Audits – Growing emphasis on bias detection and fairness assessments to ensure responsible AI use.
Summary
Model Risk Management is a critical discipline that ensures models are reliable, transparent, and compliant with regulatory standards. Organizations must implement a structured governance framework, conduct rigorous model validation, and stay abreast of emerging trends to mitigate model risks effectively. By adopting best practices and leveraging technology, businesses can enhance decision-making while minimizing risks associated with model failures.
With regulatory scrutiny increasing and AI-driven models becoming more prevalent, a proactive approach to MRM will be essential for organizations to maintain trust, efficiency, and compliance in the evolving landscape.