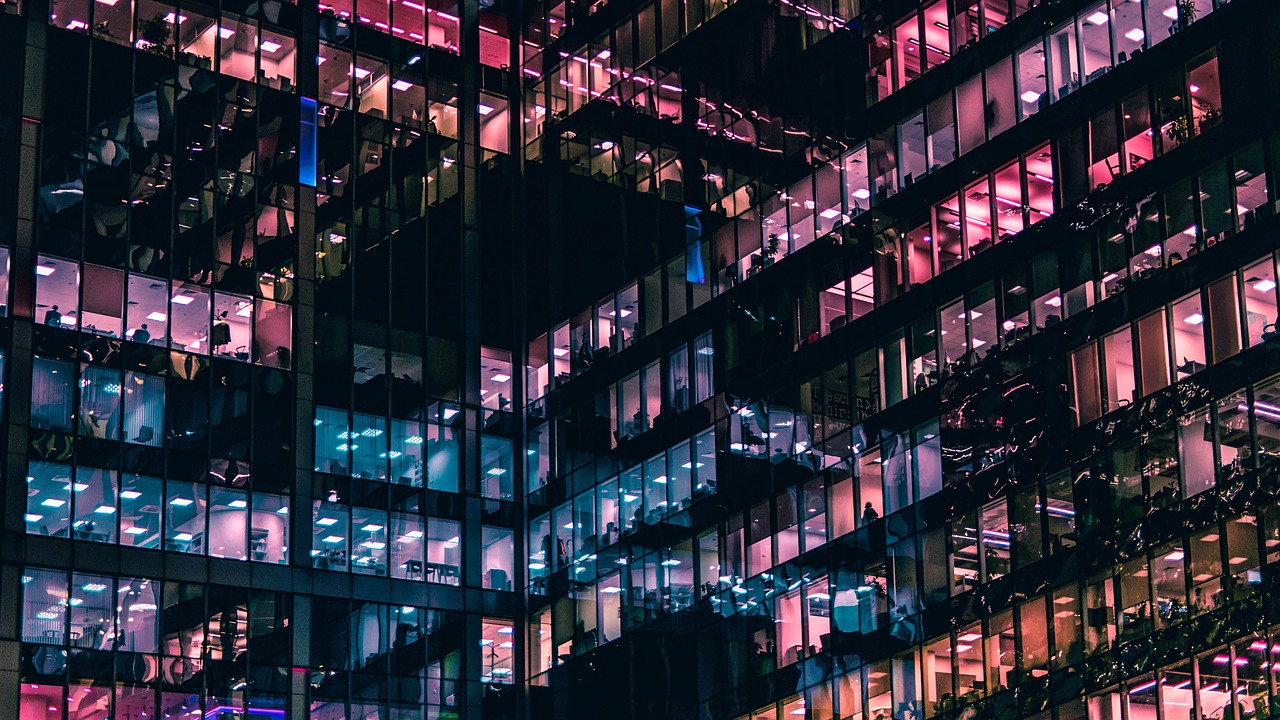
Introduction
In an increasingly digital world, organizations face a variety of risks ranging from financial uncertainties to cybersecurity threats. Effective risk management relies on accurate data and advanced analytical tools to predict, mitigate, and respond to risks efficiently. Artificial Intelligence (AI) has emerged as a transformative force in risk management, providing sophisticated models and predictive capabilities that enhance decision-making processes. However, the integration of AI in risk management necessitates a high level of data transparency to ensure accuracy, accountability, and ethical governance. This paper explores the critical role of data transparency in AI-driven risk management, highlighting its implications, challenges, and best practices.
The Role of AI in Risk Management
AI-driven risk management involves the use of machine learning (ML), natural language processing (NLP), and predictive analytics to assess and mitigate potential risks. AI systems can process vast amounts of structured and unstructured data, identifying patterns and anomalies that traditional methods may overlook.
Key AI Applications in Risk Management:
Fraud Detection: AI algorithms analyze transactional patterns to detect fraudulent activities in banking, insurance, and e-commerce.
Cybersecurity: AI monitors network activity, identifying potential threats and vulnerabilities.
Financial Risk Analysis: Predictive analytics assess credit risk, market volatility, and liquidity risks.
Supply Chain Risk Management: AI evaluates supplier performance and external factors affecting supply chains.
Operational Risk Assessment: AI detects inefficiencies, compliance issues, and internal risks within organizations.
Despite its advantages, AI’s effectiveness in risk management depends significantly on data quality, accessibility, and transparency.
Understanding Data Transparency
Data transparency refers to the availability, accuracy, and comprehensibility of data used in decision-making processes. In AI-driven risk management, data transparency ensures that AI models function optimally, maintain accountability, and align with regulatory standards.
Components of Data Transparency:
Data Accessibility: Risk management professionals must have access to relevant datasets for validation and interpretation.
Data Accuracy: AI systems should be trained on high-quality, bias-free, and representative data.
Explainability: Stakeholders should understand how AI models arrive at their conclusions.
Regulatory Compliance: Adherence to data protection laws such as GDPR and CCPA is essential.
Ethical Considerations: Ensuring data usage aligns with ethical standards and prevents discrimination.
The Intersection of Data Transparency and AI in Risk Management
The synergy between AI and data transparency plays a vital role in risk management, enhancing reliability, accountability, and trust. Organizations that prioritize transparency can mitigate AI biases, improve predictive accuracy, and ensure compliance with industry regulations.
Benefits of Data Transparency in AI Risk Management:
Improved Decision-Making: Transparent data enables risk managers to validate AI-driven insights.
Regulatory Adherence: Transparent AI models facilitate compliance with financial and data privacy regulations.
Bias Mitigation: Transparency helps identify and rectify biases in AI training datasets.
Stakeholder Trust: Investors, regulators, and customers gain confidence in AI-driven risk assessments.
Enhanced Collaboration: Open access to data fosters collaboration among departments and industries.
Despite these benefits, achieving full data transparency in AI remains a challenge due to data complexity, proprietary algorithms, and evolving regulatory landscapes.
Challenges in Achieving Data Transparency in AI Risk Management
Data Privacy Concerns: Organizations must balance transparency with the need to protect sensitive information.
Complex AI Models: Many AI-driven risk assessment models function as “black boxes,” making their decision-making processes difficult to interpret.
Data Silos: Fragmented data storage across departments hinders transparency and accessibility.
Regulatory Variability: Different regions and industries have varying transparency and data-sharing regulations.
Bias and Ethical Dilemmas: AI systems may unintentionally reinforce biases if trained on unbalanced datasets.
To address these challenges, organizations must adopt strategic frameworks and best practices for integrating AI with transparent risk management.
Best Practices for Enhancing Data Transparency in AI Risk Management
1. Adopting Explainable AI (XAI)
Explainable AI techniques provide insights into how AI models generate predictions. Methods such as SHAP (Shapley Additive Explanations) and LIME (Local Interpretable Model-agnostic Explanations) help risk managers understand AI decisions.
2. Ensuring Robust Data Governance
Establishing strong data governance frameworks ensures that data used in AI models is accurate, unbiased, and ethically sourced. Key measures include:
Data validation and cleansing
Regular audits of AI systems
Defining clear data ownership and accountability
3. Complying with Regulatory Standards
Organizations should align AI risk management practices with regulatory frameworks such as:
General Data Protection Regulation (GDPR)
California Consumer Privacy Act (CCPA)
Basel III and IV for financial risk compliance
ISO 27001 for information security
4. Implementing Transparent Data Policies
Risk management teams should document and communicate data policies that outline:
Data collection and usage procedures
AI model decision-making transparency
User rights regarding data access and correction
5. Fostering Ethical AI Use
Ethical AI principles ensure fairness, accountability, and transparency. Organizations should:
Regularly audit AI models for biases
Promote diversity in AI development teams
Establish AI ethics committees
6. Enhancing Cross-Functional Collaboration
Risk management teams should work alongside data scientists, IT, legal experts, and regulatory bodies to ensure AI-driven risk management aligns with transparency standards.
Case Studies: AI and Data Transparency in Risk Management
Case Study 1: AI in Financial Risk Management
A major global bank implemented AI for credit risk assessment. Initially, customers and regulators were concerned about opaque decision-making. By adopting explainable AI techniques and transparent data policies, the bank improved regulatory compliance and customer trust.
Case Study 2: AI in Cybersecurity Risk Management
A technology firm leveraged AI to detect cyber threats. The organization enhanced transparency by providing detailed threat reports, enabling IT teams to understand and respond to AI-generated alerts effectively.
Case Study 3: AI in Healthcare Risk Management
A healthcare provider used AI to predict patient readmission risks. By implementing transparent data-sharing policies and complying with HIPAA regulations, the provider ensured ethical AI usage while improving patient care.
Future of AI and Data Transparency in Risk Management
The future of AI in risk management will see increasing regulatory oversight, advances in explainable AI, and the adoption of decentralized data-sharing models like blockchain. Emerging trends include:
AI Audits: Independent audits will ensure AI compliance and transparency.
Federated Learning: AI models trained on decentralized data improve privacy while maintaining transparency.
Blockchain for Risk Management: Immutable records enhance data integrity and accountability.
Summary
Data transparency is fundamental to the effective use of AI in risk management. Organizations that prioritize transparency can improve decision-making, mitigate biases, and enhance regulatory compliance. By adopting explainable AI, robust data governance, and ethical AI practices, businesses can harness AI’s full potential while ensuring accountability and trust. As AI continues to evolve, maintaining a balance between innovation and transparency will be essential for sustainable risk management practices in the digital age.