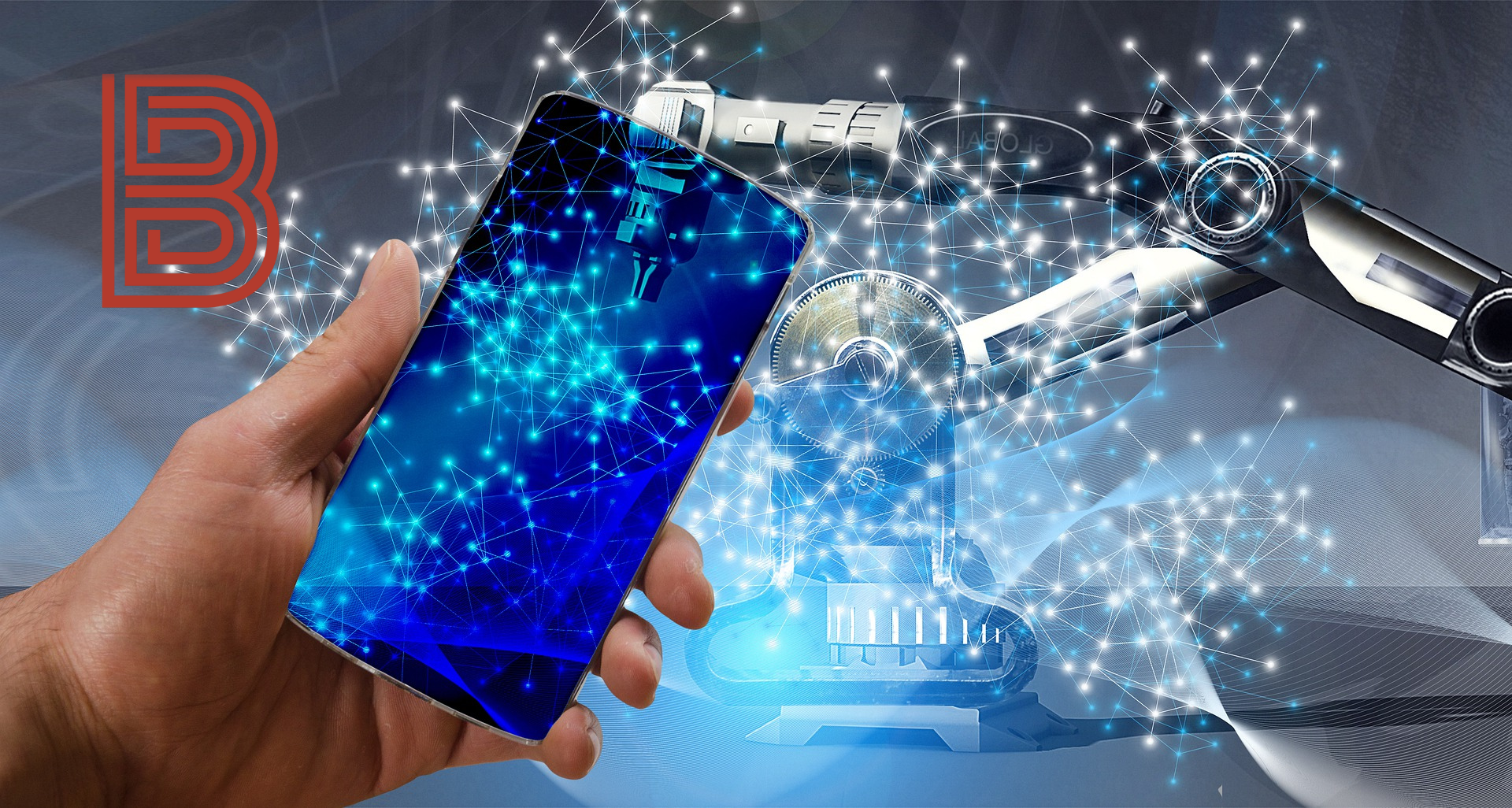
Introduction
In today’s increasingly interconnected and digitized business landscape, the reliance on third-party vendors has become not only commonplace but essential. Organizations depend on suppliers, service providers, contractors, and partners to enhance efficiency, drive innovation, and reduce costs. However, this reliance introduces significant risks, including operational, financial, compliance, reputational, and cybersecurity threats. Managing these risks effectively is crucial for organizational resilience and success. Artificial Intelligence (AI) has emerged as a transformative force in third-party risk management (TPRM), offering unprecedented capabilities to identify, assess, monitor, and mitigate risks associated with third-party relationships.
Understanding Third-Party Risk Management
Third-party risk management encompasses the strategies, processes, and tools used by organizations to identify, assess, and mitigate risks posed by external entities they engage with. These risks can stem from various areas, including data breaches, regulatory non-compliance, supply chain disruptions, and ethical concerns. The TPRM process typically includes due diligence, risk assessment, contract management, continuous monitoring, and incident response.
Traditional TPRM methods often involve manual processes, spreadsheets, and siloed data systems, which can be inefficient, error-prone, and unable to scale with the growing number and complexity of third-party relationships. As the risk landscape evolves and regulatory expectations increase, organizations are turning to AI to enhance their TPRM capabilities.
The Role of AI in TPRM
Artificial Intelligence refers to the simulation of human intelligence in machines that are capable of learning, reasoning, and decision-making. In TPRM, AI technologies such as machine learning (ML), natural language processing (NLP), robotic process automation (RPA), and predictive analytics are being leveraged to automate and improve various aspects of the risk management process.
Key Applications of AI in TPRM
Automated Risk Assessment:
AI-powered systems can automate the initial risk assessment of third parties by analyzing vast amounts of structured and unstructured data from various sources, including financial statements, news articles, regulatory filings, and social media. Machine learning algorithms can identify risk patterns and generate risk scores, enabling faster and more accurate assessments.
Continuous Monitoring:
Traditional risk assessments are often conducted periodically, which may not capture emerging risks. AI enables real-time and continuous monitoring of third parties by analyzing data streams and detecting anomalies or significant changes in risk indicators. This proactive approach helps organizations respond to potential issues before they escalate.
Enhanced Due Diligence:
Natural language processing allows AI systems to extract relevant information from legal documents, contracts, and compliance reports, reducing the time and effort required for due diligence. NLP can also identify clauses related to risk exposure, data protection, and regulatory compliance.
Predictive Analytics:
By analyzing historical data and identifying trends, AI can predict potential risks and future performance of third parties. Predictive models can forecast supply chain disruptions, financial instability, or compliance failures, enabling organizations to take preventive measures.
Cyber Risk Management:
AI can enhance cybersecurity risk management by monitoring network traffic, detecting threats, and assessing the cyber hygiene of third-party ndors. ML algorithms can identify suspicious activities and potential vulnerabilities in real-time.
Risk Categorization and Prioritization:
AI can classify third parties based on risk categories such as operational, reputational, financial, and compliance risks. It can prioritize vendors for in-depth assessments based on their criticality and risk exposure.
Regulatory Compliance:
AI tools can assist in tracking regulatory changes and assessing their impact on third-party relationships. They can also ensure that third parties comply with relevant laws and regulations, such as GDPR, HIPAA, and anti-bribery laws.
Benefits of AI in TPRM
Efficiency and Scalability:
AI automates labor-intensive tasks, reducing the time and cost associated with risk management activities. It enables organizations to scale their TPRM programs to manage hundreds or thousands of third parties effectively.
Improved Accuracy:
AI reduces human errors and subjectivity in risk assessments. Machine learning algorithms can analyze large datasets more accurately than manual methods, leading to better risk identification and decision-making.
Real-Time Insights:
Continuous monitoring and real-time analytics provide organizations with up-to-date information on third-party risks, enabling quicker and more informed responses to emerging threats.
Enhanced Risk Mitigation:
Predictive capabilities allow organizations to anticipate and prevent risks before they materialize. This proactive approach minimizes the impact of third-party failures on business operations.
Strategic Decision-Making:
AI-generated insights support strategic decision-making by providing a comprehensive view of the third-party risk landscape. Executives can allocate resources more effectively and align risk management with business objectives.
Challenges and Considerations
While AI offers significant advantages in TPRM, its implementation comes with challenges that organizations must address:
Data Quality and Availability:
AI systems rely on high-quality data to function effectively. Incomplete, outdated, or inconsistent data can lead to inaccurate risk assessments. Organizations must invest in data governance and integration to ensure data reliability.
Model Transparency and Explainability:
Many AI models, especially deep learning algorithms, are often criticized for their lack of transparency. Stakeholders may find it difficult to understand how a risk score was generated. Ensuring model explainability is crucial for trust and regulatory compliance.
Integration with Existing Systems:
Integrating AI tools with legacy TPRM systems and workflows can be complex. Organizations need to ensure seamless interoperability and minimal disruption to existing processes.
Ethical and Legal Concerns:
The use of AI raises ethical and legal questions, particularly related to data privacy, bias, and accountability. Organizations must implement ethical AI practices and comply with relevant data protection regulations.
Change Management:
Adopting AI requires cultural and organizational change. Employees may resist automation due to fear of job loss or unfamiliarity with new technologies. Effective change management and training are essential for successful implementation.
Case Studies and Industry Examples
Financial Services:
Banks and financial institutions use AI-driven platforms to assess the risk of third-party vendors, particularly in areas like anti-money laundering (AML) and fraud detection. AI helps identify suspicious transactions and flag high-risk vendors for further investigation.
Healthcare:
Healthcare organizations rely on AI to evaluate the compliance and data security practices of third-party service providers, especially those handling sensitive patient information. NLP tools assist in reviewing contracts and ensuring HIPAA compliance.
Manufacturing:
Manufacturers use predictive analytics to assess the stability of suppliers and prevent supply chain disruptions. AI models forecast potential delays based on geopolitical events, natural disasters, and financial indicators.
Technology Sector:
Tech companies leverage AI to monitor the cybersecurity posture of their vendors. Machine learning algorithms analyze network behavior and detect vulnerabilities in third-party software integrations.
Future Trends and Outlook
The role of AI in third-party risk management is expected to grow as organizations seek to enhance resilience and agility in an increasingly complex risk environment. Future developments may include:
AI-Powered Risk Intelligence Platforms:
Integrated platforms that combine AI, big data, and blockchain technologies will offer a holistic view of third-party risks. These platforms will enable end-to-end risk management and decision support.
Advanced Natural Language Understanding:
Improvements in NLP will enhance the ability of AI systems to interpret complex legal language and contractual obligations, further automating due diligence and compliance tasks.
Collaborative AI Models:
Federated learning and collaborative AI models will allow organizations to share risk intelligence without compromising data privacy. This collective approach can enhance the accuracy and coverage of risk assessments.
Ethical AI and Governance:
There will be increased focus on developing ethical AI frameworks and governance structures to ensure responsible use of AI in risk management. Regulatory bodies may introduce guidelines specific to AI-driven TPRM.
Human-AI Collaboration:
AI will augment rather than replace human decision-makers. Risk managers will use AI-generated insights to make informed judgments, combining machine efficiency with human expertise.
Summary
Artificial Intelligence is revolutionizing third-party risk management by offering innovative tools and techniques to identify, assess, and mitigate risks more effectively. While challenges remain, the benefits of AI-driven TPRM are substantial, including improved efficiency, accuracy, and strategic insight. As technology continues to evolve, organizations that embrace AI responsibly and thoughtfully will be better positioned to navigate the complexities of third-party relationships and safeguard their operations in an unpredictable world.
By integrating AI into their risk management frameworks, businesses can not only enhance their resilience but also gain a competitive edge in a landscape where third-party risks are more significant and multifaceted than ever before.