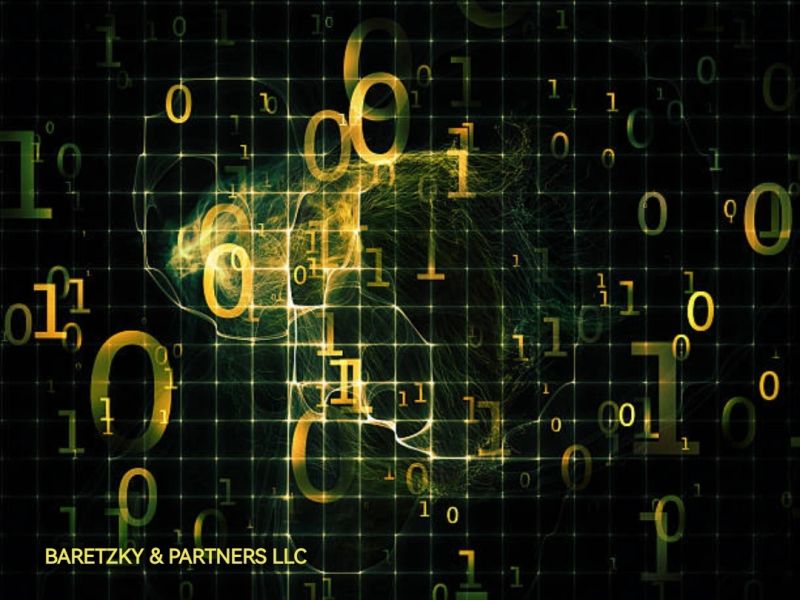
A Markov chain is defined by its memoryless property, meaning the future state of the system depends only on the current state, not on the path taken to reach it. This simplicity makes Markov chains a powerful framework for modeling dynamic systems in finance, insurance, operations, and more.
In risk management, Markov chains can be applied to model various processes, such as credit ratings, insurance claims, and asset prices. For example, in credit risk, a company or individual’s credit rating can be modeled as a Markov process, where the likelihood of transitioning from one credit rating to another (e.g., from AA to A) is defined by transition probabilities. These probabilities are derived from historical data, allowing risk managers to assess the chances of default or downgrade over time.
In insurance, Markov chains can be used to estimate the frequency of claims or the likelihood of policyholders moving between different risk categories. This helps insurers set premiums more accurately and prepare for potential future liabilities.
Markov chains also enable scenario analysis. By simulating various states and transitions, companies can stress-test their portfolios or operations under different conditions, assessing potential losses or disruptions. Additionally, Markov models help quantify and price risk, making them vital in risk management strategies such as Value-at-Risk (VaR) calculations or operational risk modeling.
WWW.BARETZKY.NET